Artificial intelligence for the histological prediction of ulcerative colitis remission
In a recent study published in Gastroenterology, researchers assessed the effectiveness of artificial intelligence in the histological prediction of ulcerative colitis remission or clinical outcomes.
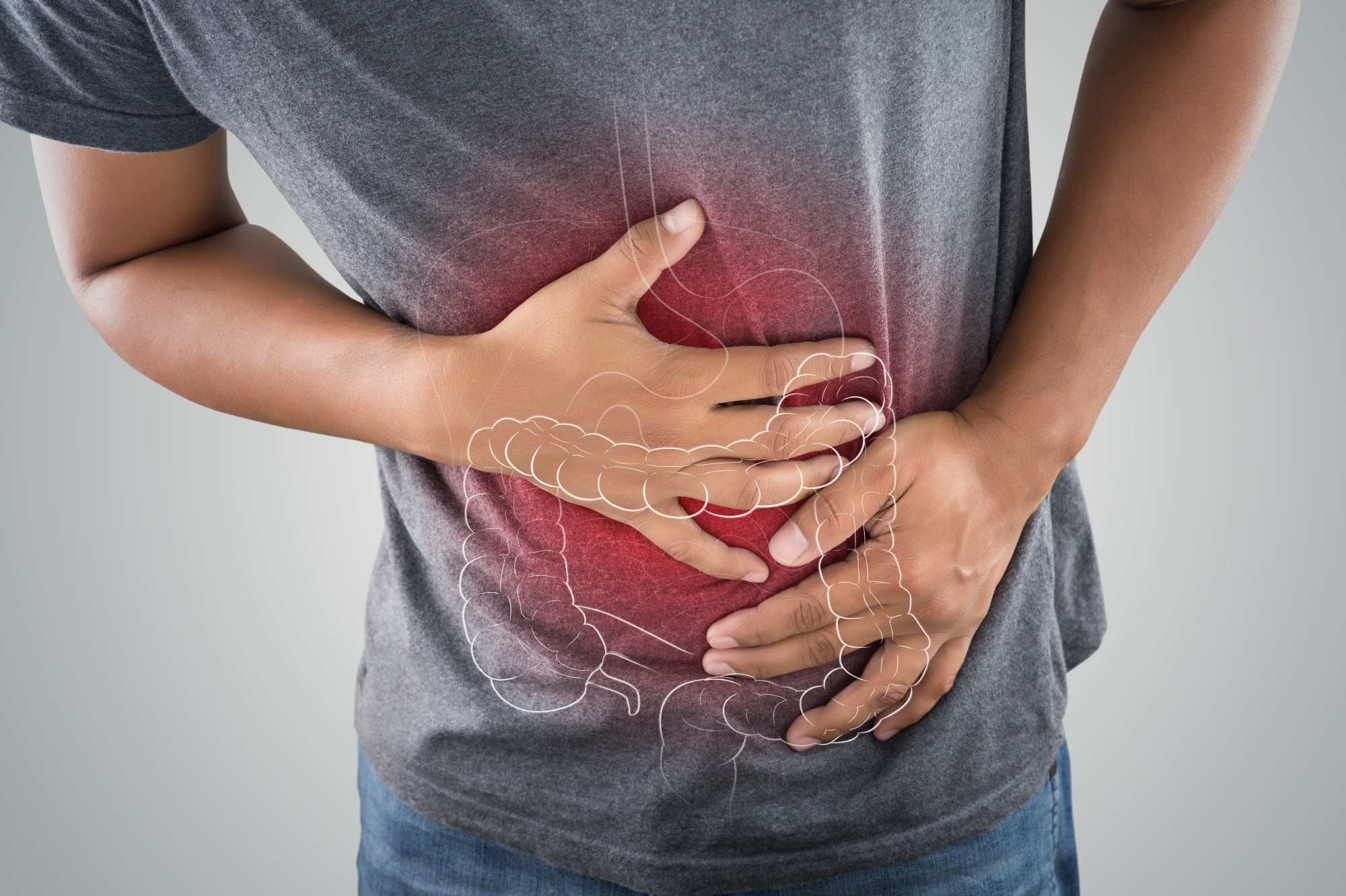
Background
Ulcerative colitis (UC) is a remitting and relapsing chronic inflammatory bowel disease (IBD). To prevent complications, UC treatment aims to eliminate inflammation, with histopathology being the most precise way for detecting inflammation and distinguishing it from remission. Histologic remission (HR) is associated with better clinical results and has thus become a therapy objective. Artificial intelligence (AI)-based computer-aided diagnostic (CAD) systems are widely employed to simplify and standardize the interpretation of medical imaging. These tools can potentially improve examination, facilitate interpretation, and eliminate disagreements among pathologists.
About the study
In the present study, researchers sought to build and validate an AI-aided CAD system for evaluating UC samples and predicting prognosis.
From September 2016 to November 2019, the team recruited patients from 11 centers for the primary analysis. The eligible participants had confirmed UC for over a year, irrespective of disease activity and a colonoscopy indication. A minimum of two targeted tissue specimens were obtained from the most typical sites of healing or inflammation present in the rectum and sigmoid, which were the same regions where the endoscopic evaluation was recorded.
For prognosis assessment, the clinical outcomes associated with UC-related hospital admission, UC-related surgery, and increase or variations in UC therapy initiation, such as steroids, biological agents, and immunomodulators, were selected as surrogates for disease flare. These proxies were recorded via follow-up phone visits or calls conducted 12 months after endoscopy within the initial group or up to 33 months within the external validation group.
Picasso Histologic remission index was implemented within the CAD system by devising a framework constructed according to multiple-instance learning with restrictions. The final whole-biopsy prediction was derived using a multiple-instance learning strategy that assessed the examination of each biopsy patch and combined the assessment into a final result, activity, or remission as per PHRI. The diagnostic performance of the CAD was estimated as sensitivity (SE), negative predictive value (NPV), positive predictive value (PPV), specificity (SP), F1-Score (F1S), area under the receiver operating characteristic curve (AUROC), and accuracy (ACC).
Results
Initially, a total of 535 samples were utilized for model development and testing. These samples were obtained from 273 individuals, of which 40.7% were female, and the average age was 48.1 years. Depending on the criteria used to evaluate them, between two-thirds and three-quarters of biopsies were histologically in remission. From the initial 535 biopsies, almost 118 were employed for model training, 42 for model calibration, and 375 for its validation. Thereafter, 154 more samples from 58 UC patients were utilized for external validation. In a validation set of 375 biopsies, the team noted that the CAD system differentiated histologic remission from disease-related activity as defined by PHRI with 89% sensitivity, 85% specificity, 75% PPV, 94% NPV, 87% accuracy, and an AUROC of 87%.
The same method trained to identify neutrophils and predict PHRI was then compared to human evaluations of remission/activity based on the Robart Histopathology index (RHI) and Nancy Histological index (NHI). The team observed that the system distinguished histological remission and activity with 94% sensitivity, 76% specificity, 53% PPV, 98% NPV, 80% accuracy, and an AUROC of 85%. On the other hand, the CAD system revealed 89% sensitivity, 79% specificity, 60% PPV, 95% NPV, 81% accuracy, and an AUROC of 86%. Notably, the AUROC differences were not statistically significant.
When patients in histological activity or remission were categorized based on pathologists' evaluation, the team observed that the hazard ratios between the group experiencing any pre-specified adverse clinical event and the group with a surrogate for a flare-up, was 3.56 as per PHRI, 4.28 as per RHI, and 3.56 according to NHI. However, when the same assessment was conducted by the CAD system which was trained to differentiate between PHRI activity and remission, the hazard ratio was 4.64, which was comparable to and numerically greater than that performed by human experts taking into account any of the scores analyzed.
Overall, the study findings showed that the CAD system employed in the study accurately differentiated disease remission from disease activity and provided a good forecast of the accompanying endoscopic activity as well as the risk of flare. The researchers believe that further developments will involve the detection of dysplasia and the integration of histologic and endoscopic AI models into a unified disease monitoring and prediction tool.
- Iacucci M, Parigi TL, del Amor R, Meseguer P, Mandelli G, Bozzola A, Bazarova A, Bhandari P, Bisschops R, Danese S, De Hertogh G, Ferraz JG, Goetz M, Grisan E, Gui X, Hayee B, Kiesslich R, Lazarev M, Panaccione R, Parra-Blanco A, Pastorelli L, Rath T, Røyset ES, Tontini GE, Vieth M, Zardo D, Ghosh S, Naranjo V, Villanacci V. (2023). Artificial Intelligence enabled histological prediction of remission or activity and clinical outcomes in ulcerative colitis. Gastroenterology (2023). doi: https://doi.org/10.1053/j.gastro.2023.02.031 https://www.gastrojournal.org/article/S0016-5085(23)00216-0/fulltext
Posted in: Medical Science News | Medical Research News | Disease/Infection News
Tags: Artificial Intelligence, Biopsy, Chronic, Colonoscopy, Diagnostic, Dysplasia, Endoscopy, Gastroenterology, Histopathology, Hospital, Imaging, Inflammation, Inflammatory Bowel Disease, Medical Imaging, Neutrophils, Surgery, Ulcerative Colitis
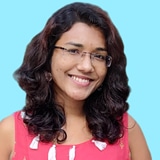
Written by
Bhavana Kunkalikar
Bhavana Kunkalikar is a medical writer based in Goa, India. Her academic background is in Pharmaceutical sciences and she holds a Bachelor's degree in Pharmacy. Her educational background allowed her to foster an interest in anatomical and physiological sciences. Her college project work based on ‘The manifestations and causes of sickle cell anemia’ formed the stepping stone to a life-long fascination with human pathophysiology.
Source: Read Full Article