A non-mechanistic approach to real-time forecasting of U.S. COVID-19 pandemic
The coronavirus disease 2019 (COVID-19) pandemic is caused by the outbreak of the severe acute respiratory syndrome coronavirus 2 (SARS-CoV-2). The current pandemic has shown the importance of accurately forecasting infection and mortality rates to inform public health strategies.
However, there exists a plethora of modeling techniques that are associated with their own respective advantages and disadvantages. A new study published on the medRxiv* preprint server introduces the non-mechanistic MIT-LCP forecasting model and compares its performance to various other models that have been proposed for forecasting COVID-19 dynamics.
Study: Forecasting the COVID-19 Pandemic: Lessons learned and future directions. Image Credit: everything possible / Shutterstock.com
Background
The United States Centers for Disease Control and Prevention (CDC) invited an open call for models to forecast COVID-19 cases and deaths at the state level. This led to the organic creation of groups consisting of individuals with varied backgrounds and expertise, as well as a shared vision of forecasting to inform public policy. Soon, fault lines were highlighted with regard to the inability of one group (research team, university, or country) to harness the power of data to understand and forecast the trajectory of the pandemic.
Forecasting models typically fall along a spectrum of ‘mechanistic’ to ‘non-mechanistic models. Mechanistic models incorporate a given understanding of the underlying causal structure of the data generation process, while the latter does not embed such structural assumptions.
The approach used by the scientists of this study employs standard machine learning techniques that impose little structure on the data distribution (non-mechanistic). The results of this model provide a benchmark against which to measure mechanistic models.
About the study
Non-mechanistic approaches have been utilized in numerous applications including influenza forecasting, and population dynamics of beetles, to name a few. During the Ebola epidemic, flexible non-mechanistic or semi-mechanistic models were used since parameterizing mechanistic models were often difficult in real-time. In the past, non-mechanistic models have had promising results in real-time forecasting when compared to traditional statistical models.
To implement the model, the researchers of the current study used a gradient boosted regressor to forecast COVID-19 deaths at state and national levels. Novel digital data sources including prior COVID-19 cases and deaths, as well as demographic, socioeconomic, mobility data were used. These variables were valuable, as certain groups of people are more vulnerable to COVID-19.
The Johns Hopkins University Center for Systems Science and Engineering (JHU CSSE) deaths and cases data was used to train the model, whereas 3-week lagged cases and deaths were used to predict 1-4 weeks ahead.
In terms of model diagnostics, the R2 score (Coefficient of Determination) was used in the training of the model. The objective was to maximize the R2 score. Forecast Absolute Percentage Error (FAPE) was used for the national-level comparison, while Mean Absolute Error (MAE) was used for the state-level comparison.
Study findings
To evaluate the MIT-LCP model performance, seven other CDC Forecast Hub models were chosen for comparison. The model reported a lower median FAPE compared to four out of five mechanistic SEIR models.
Furthermore, the MIT-LCP model achieved a median FAPE of 15.05%, at the national level, across the 22 weeks for all forecast dates. Most SEIR models were in the range of 20-25%. The MIT-LCP model also had a smaller interquartile range within the distribution of FAPEs as compared to most mechanistic models.
With respect to the U.S. state mortality forecast, the MIT-LCP model had a lower median MAE compared to most mechanistic models for one week ahead of target. This subsequently improved over most models.
The researchers documented significant variability in the distribution of errors by forecast date, which highlights the difficulty in forecasting throughout the pandemic.
Limitations
One important limitation of non-mechanistic models is the lack of causal inference. More research is required to integrate causal inference methods with machine learning in non-mechanistic models.
A second limitation is the lack of policy data integration with forecasting models. With the wider availability of policy datasets, scientists could standardize conditional predictions. This would substantially enhance the accuracy of forecasts and expand the potential impact of the forecasts on policy decisions.
Conclusion
Forecasts about the trajectory of infectious diseases provide critical data for informing public health policy and interventions. Mechanistic and non-mechanistic forecasting models each have their own respective advantages and disadvantages.
Besides introducing the MIT-LCP model, this study calls attention to the organically growing community of data experts spanning multiple disciplines who have a shared purpose of harnessing the power of big data to forecast the future trajectory of the pandemic. This is absolutely crucial to help governments and policymakers devise appropriate strategies to contain the current and future pandemics.
*Important notice
medRxiv publishes preliminary scientific reports that are not peer-reviewed and, therefore, should not be regarded as conclusive, guide clinical practice/health-related behavior, or treated as established information.
- Sundar, S., Schwab, P., Tan, J. Z. H., et al. (2021) Forecasting the COVID-19 Pandemic: Lessons learned and future directions. medRxiv. doi:10.1101/2021.11.06.21266007. https://www.medrxiv.org/content/10.1101/2021.11.06.21266007v1
Posted in: Device / Technology News | Medical Research News | Disease/Infection News
Tags: Coronavirus, Coronavirus Disease COVID-19, Diagnostics, Infectious Diseases, Influenza, Machine Learning, Mortality, Pandemic, Public Health, Research, Respiratory, SARS, SARS-CoV-2, Severe Acute Respiratory, Severe Acute Respiratory Syndrome, Syndrome
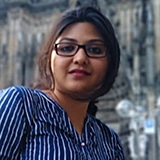
Written by
Dr. Priyom Bose
Priyom holds a Ph.D. in Plant Biology and Biotechnology from the University of Madras, India. She is an active researcher and an experienced science writer. Priyom has also co-authored several original research articles that have been published in reputed peer-reviewed journals. She is also an avid reader and an amateur photographer.
Source: Read Full Article